Background
The MeToo movement does not exist without social media, especially Twitter. Ever since october 2017, women from various parts of the world started breaking their silence about past misconducts they suffered, trying to hold personnalities accountable (mostly male politicians and entertainment celebrities). The movement transcended virtual spaces and went to the streets. Women are not objects, and sexual harrasment should be talked about. The activists' goals are simple. Not only should the past be dug up, but one should not be afraid to speak up and fight for what is right.
As we can see in the chart below taken from Google trends, the emergence of MeToo (it did not exist before Alyssa Milano wrote her famous tweet) created a wave of searches around the topic of sexual harrasment, which made it peak at the maximal index of interest during the last week of November 2017. The index then came back to where it was before, signifying that the MeToo movement might not have a large long-term influence outside of social media.Which is why we will try to answer these questions:
Did MeToo have an impact on the place of women in media? And if so, was it all positive, or did it have negative repercussions?
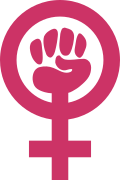
Women speak much less than men.
After a first look at the data, we can see that the percentage of women speakers in the whole dataset varies between 15 and 20%. We also see that starting from late 2017, the percentage starts going up slightly, which coincides with the first Metoo tweet. We deduce then that MeToo gave more place to women in media, and our study is done...
It is of course not that simple, and even though the rise in percentages is statistically significant, we cannot draw any conclusions this early yet.